NEWS
We’re living in the data-driven era of IT where information can almost be recognized as the new international currency. Proper data management is vital for organizations big and small, but the challenges continue to grow.
With data getting more complex, diverse, and exponentially voluminous, old-school solutions to managing it just won’t cut it. Meeting the overwhelming demands of modern technological and business landscapes requires evolved ways of approaching data management.
In this article, we’re exploring just that — understanding apparent trends and ways in which technology enables institutions to harness their informational assets. So without further ado, let’s have a glance into the future of data management.
1. The Rise of Data Lakes and Data Fabrics

In modern data architecture, data lakes play a significant role. They store data in its most raw form and its native format. This is contrary to traditional data archives where data must be organized and structured as required before storing it. This subtle difference adds lots of flexibility in the way businesses or institutions gather and store data, regardless of how diverse the sources may be. For instance, it could be captured from social media, logs, IoT devices, or even surveys — void of any preprogrammed schemas.
Data fabrics are also rising in trend which can be used to seamlessly handle data that’s distributed across cloud-based or on-premises infrastructures. Providing a simplistic view that unifies all informational assets, data fabrics allow easy and holistic management, analysis, and control of data.
2. Artificial Intelligence (AI) and Machine Learning (ML) for Data Management

AI has grown immensely over the last couple of years, and its implications in the digital realm of data management are game-changing. Data management practices are getting revolutionized by machine learning and AI, and it’s only going to get better since we’re still in the early stages of seeing what artificial intelligence can do. Still, AI-powered tools have already achieved automation in complex management tasks like data duplication, indexing, classification, and cleansing.
Machine-learning models can also perform accurate analysis of patterns to predict future trends based on historical data. In addition to optimized retrieval and storage of data, security is also enhanced with ML models as they can identify behavioral anomalies effectively.
Moreover, data governance solutions that are backed by AI make it much easier for companies to ensure regulatory compliance. They help maintain the integrity and quality of data by mitigating security or malpractice risks across the board.
As these models continue to advance, they’re projected to streamline and optimize data management even more. However, AI is not where it can be right now, so while there are many pros to its assistance, there are also some cons that may fade with time.

3. DataOps: Collaborative Data Management Practices

DataOps practices focus on boosting agility, automation, efficiency, and collaboration in the data management workflows of an organization. By promoting the adoption of quicker and more effective methodologies, deployment practices, and continuous integration, DataOps maintains reliability and quality while also accelerating the processing and delivery of insights driven by data.
Thanks to “data pipelines” — a concept integral to DataOps — the flow of data can be automated from its source, all the way to its consumption. Every part of the process including validating data, transforming it, and its monitoring falls under the umbrella of DataOps.
It breaks silos down between data science, engineering, and IT operators, fostering an innovative and collaborative culture within an organization. This sort of environment is exactly what most businesses need to thrive and promptly tend to enterprise needs in today’s digital age.
4. Edge Computing and Edge Data Management

IoT devices have proliferated faster than ever over the last few years, and that has called for an increased demand for real-time analytical data. As a decentralized paradigm of computing, ‘edge computing’ has quickly gained popularity as a solid solution.
By processing the data when it is closer to its source (or at the network’s “edge” — hence the name), the system does not need to rely on cloud infrastructures or centralized data centers.
Partnering up with edge computing, we also have edge data management solutions for addressing the all-new problems related to data management near the source. Some such challenges are intermittent connectivity, limited available bandwidth, the need for very low latency, etc.
With edge computing and edge data management solutions working together, organizations can locally gather, store, secure, and analyze data. They can leverage it to quickly collect meaningful data-driven insights and act on them. The benefits of centralization also need not be sacrificed as edge data management platforms also offer mechanisms to aggregate and synchronize data with centralized repositories.
5. Blockchain for Secure and Immutable Data Management

Impenetrable security is non-negotiable when it comes to the management of data. Even people unaware of the IT jargon understand this today and keep antivirus software on their iPhones. So, it only makes sense for companies to look for cutting-edge security solutions like blockchain.
Most of us have heard the term “blockchain” by now due to the key role of this technology in crypto. Of late, it’s also under increasing exploration for the potentially significant role it can play in revolutionizing data management in terms of security.
With blockchain’s tamper-evident and decentralized ledger, transactions are linked cryptographically and stored securely across a distributed node network. With this level of immutability and transparency innate to its nature, blockchain is ideal for digital scenarios where qualities like audibility, trust, and data integrity are paramount.
Within the context of data management, blockchain technology can help establish an auditable and verifiable data lineage record, authenticating data from its source throughout the end of its lifecycle.
On top of that, smart contracts based on blockchain can facilitate secure P2P data transfer, enforce controls over data access, and automate data exchange agreements, hence reducing reliance on financial intermediaries. It also boosts privacy and security to a whole other degree.
Conclusion
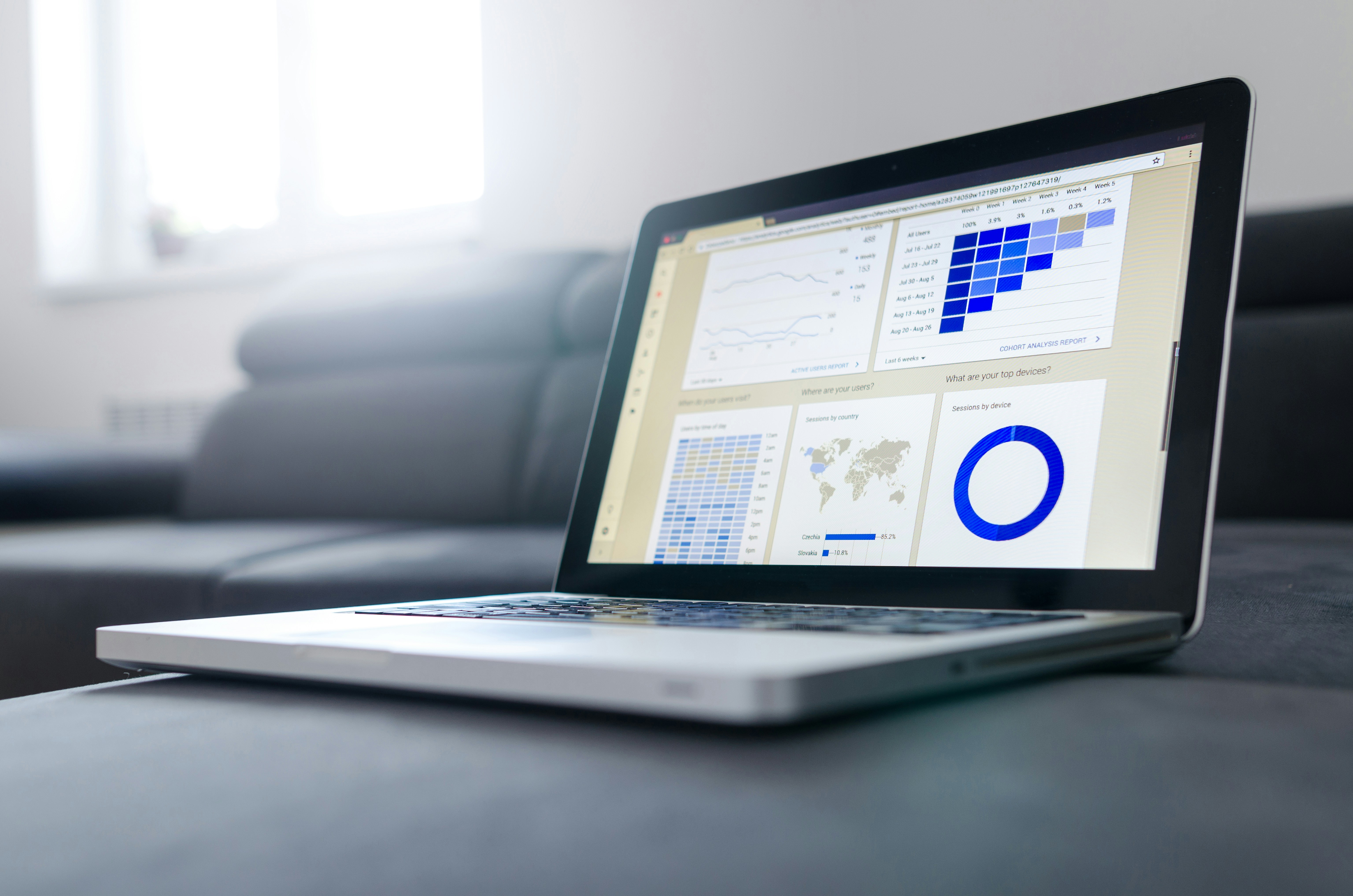
As we advance further into the digital age, the challenges in the world of IT and data management also grow — both in terms of volume and complexity. But thankfully, the solutions also lie in the same plane, and the future of data management unquestionably rests with the emerging technological trends.
From exploiting the countless benefits of data lakes to harnessing the miracles of AI and machine learning, organizations are leveraging all available digital tools to make full use of their data assets.
Organizations must stay abreast of these
rapidly evolving dynamics and embrace a collaborative and innovative culture. By doing so, they can efficiently and effectively navigate the modern data landscape with a competitive edge.
Author Bio
Fareed A. Siddiqui is an experienced researcher and writer focusing on IT topics. With 13+ years of experience as an author, he has contributed to various articles and research papers, offering valuable insights into technology trends.